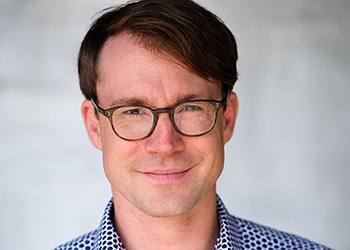
Dr. Marc Ryser is an expert in mathematical and statistical modeling. His research leverages biologic, clinical, and population-level data to inform and guide cancer early detection and prevention.
What is the role of decision aids in doctor/patient conversations?
I think evidence-based decision aids play an important role in the shared doctor/patient decision-making process. They can help patients identify their personal preferences and weigh the benefits and harms of different management options. Combined with the physician’s experience and advice, they provide a good starting point for shared decision-making.
Decision support tools are becoming increasingly sophisticated, often relying on AI algorithms. People may wonder if such tools can replace the physician, and I personally don’t think that’s going to happen anytime soon. For certain diagnostic aspects, such as finding cancers on mammograms or pathology slides, AI can be quite good, and sometimes as good as human experts. While physicians are increasingly incorporating such tools in their daily practice, I don’t think that they will become redundant in any way. Physicians will always play a critical role in interpreting modeling results and answering patients’ questions, and tools just can’t do these tasks.
What are the advantages and disadvantages of using mathematical modeling to predict cancer incidence and progression?
Mathematical modeling enables us to synthesize existing data sets in an objective, quantitative way to come up with the best possible prediction of a person’s cancer risk. Modeling is a powerful tool that helps researchers learn from what has happened in the past to then predict the future. As with everything, there are caveats to mathematical modeling. For instance, for good predictions, you not only need a good model but high-quality data. And even the best model can’t overcome serious biases in the underlying data.
To ensure the model predictions are generalizable, the data should include a diversity of races, age groups, backgrounds, and co-morbidities—essentially representing a wide expanse of populations. This isn’t always easy because most high-quality data comes from randomized clinical trials, which typically don’t represent the wider community. On the flip side, observational studies can capture more diverse populations, but the data is usually of lower quality, isn’t randomized, and may have biases and confounding. As you can see, there’s definitely a trade-off.
On cancer screening, you prefer a harm/benefit balanced approach rather than mass screening. Why is this so?
The rationale for cancer screening is that if we have a test that finds cancer early, then we can treat early and save lives. With many cancer types, we’ve been able to demonstrate the benefits of screening by seeing more early-stage and fewer late-stage cancers, as well as lower cancer mortality in screened populations compared to unscreened populations. Over time, though, we’ve realized that there is also a potential harm in screening, particularly when we overdiagnose. Overdiagnosis means that we detect and treat indolent, early-stage tumors that would not have caused symptoms or death had we not found them in the first place. And overdiagnosed patients are subject to invasive treatments and their side effects without deriving any benefit. Unfortunately, it’s difficult to quantify the degree of overdiagnosis for a given screening approach. Here’s an example: a woman undergoes mammography, is diagnosed with and treated for early-stage breast cancer, and is alive and well 20 years later. But what if the tumor was actually indolent and would not have become life threatening had we never found it by screening? Could we have saved her from losing a breast and from going through the psychological effects of receiving a cancer diagnosis? For each patient, you’ll never know if the cancer will be lethal or completely indolent. So most patients are treated aggressively to be on the safe side, and we conclude that everything worked well because we see success, which in this case is the fact that the woman is alive 20 years after her diagnosis.
In my research, I use statistical modeling tools to estimate the extent of cancer overdiagnosis due to screening. I’m definitely not opposed to population-based screening—in many instances it works well, for example in cervical cancer screening. However, I do think that it’s also important to look at the harms of screening, not just the benefits. And because I like challenges, I’m particularly interested in quantifying overdiagnosis, which poses a tricky modeling problem.
When presented with the evidence that not treating certain tumors is a viable option, there is naturally pushback from parts of the medical community. But if we can show with data that in some instances doing nothing is equivalent to doing something, we can help patients avoid the potential harms of invasive cancer treatments.